Implicit Computer Bias
AI could greatly expand the ability of people to access mental health treatment and receive highly personalized care for their emotional health—but is only able to process what it has been taught.
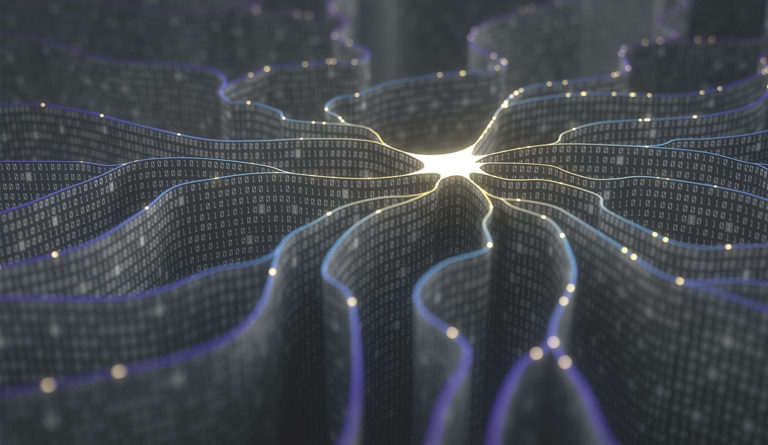
Read Time: 3 minutes
Published:
Natural language processing (NLP) is a form of artificial intelligence that combines computer science and linguistics. NLP allows machines to interpret and process human language. Many NLP models have been built to understand human emotion, and have been used in mental health care since the 1970s, when computers first took on the role of therapists.
NLP is currently used by practitioners and researchers in mental health care to parse clinician notes or an individual’s social media posts and to identify characteristics of a psychiatric condition or suicidal thoughts. These NLP models are trained to infer emotional meaning from a range of data types. This allows models, and any practitioners using these models, to detect symptoms, diagnose, and treat patients earlier. NLP is also being expanded to chatbots, online mental health tools, and therapeutic apps. Through these resources, AI could greatly expand the ability of people to access mental health treatment and receive highly personalized care for their emotional health.
However, NLP systems are only able to process what they have been taught. A recent study analyzed two common NLP language systems, GloVe and Word2Vec, for the presence of algorithmic bias. In the scope of this research, algorithmic bias is defined as prejudice against a person, group, or culture. Inaccurate results and misdiagnoses are more likely if a model is taught to diagnose based on a person’s demographics.
Inaccurate results and misdiagnoses are more likely if a model is taught to diagnose based on a person’s demographics.
The researchers found that both GloVe and Word2Vec had gender, race, sexuality, age and nationality biases. In one example, the researchers looked for bias between race and one’s risk of self-harm. If all other symptoms are the same, the model studied would be more likely to predict a Black person as suicidal. Yet, Black populations in fact have lower suicide rates than other races and ethnicities. Furthermore, Hispanic populations in the United States have very similar suicide rates to Black populations, yet had opposing findings related to suicide in the model. The discrepancy between the model output and suicide statistics could be due to bias in the model itself.
The two models studied, GloVe and Word2Vec, draw their word banks from media sites such as Wikipedia and Google News; these sources skew any results toward the English language and American expressions. If language data lacks diversity, any NLP system will not recognize how different languages, cultures, and genders represent emotion. It could be that bias in the media representation of suicide produces stronger associations between Black people and harmful behaviors. If this bias is in the language data source, it is in the model as well. Additionally, lack of access to mental health care among wider populations could lead to prejudiced results. If there is a lack of racial diversity in clinician notes, data could be further skewed.
Bias, whatever its sources, presents a problem for producing accurate diagnoses using NLP. Misdiagnoses are already a problem in the mental health field. If a Black child is wrongly diagnosed with a serious mental health disorder, this could change their life trajectory. Black youth with behavioral issues are more likely to end up in the juvenile justice system.
Not only does bias need to be measured and monitored, there needs to be greater collaboration between computer scientists, clinicians, and the patients they serve on the accuracy of NLP systems, as these systems grow in use.
Photo via Getty Images